By applying data analysis techniques used by neuroscientists to a simple man-made neural network — an Atari 2600 running “Donkey Kong” — a team of researchers found they may relay an incomplete image of how our brains work.
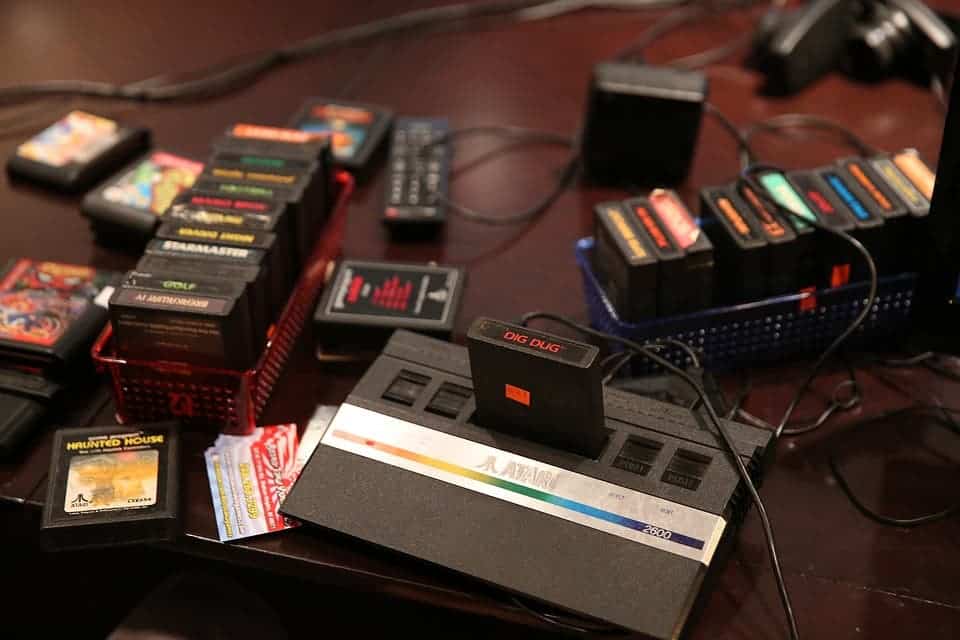
Neuroscientists today have tools at their disposal that the field could only dream about a few decades earlier. They can record the activity of more neurons at a time with better resolution than ever before. But while we can record a huge volume of data, we have no way of testing the validity of the results because we just don’t understand how even the simplest brain works.
So Eric Jonas of U.C. Berkeley and Konrad Kording of Northwestern University set out to put these algorithms to the test using a neural framework we do understand: a 6502 microprocessor housed in the Atari 2600 console.
“Since humans designed this processor from the transistor all the way up to the software, we know how it works at every level, and we have an intuition for what it means to ‘understand’ the system,” Jonas says.
“Our goal was to highlight some of the deficiencies in ‘understanding’ that arise when applying contemporary analytic techniques to big-data datasets of computing systems.”
The duo applied standard neuroscience techniques to analyze the hardware’s functions. They wanted to see how well they could re-create known characteristics such as the chipset’s architecture or the effect of destroying individual transistors. And it didn’t go very well. These techniques didn’t relay as much information about the processor as a typical electrical engineering student is expected to posses.
“Without careful thought, current big-data approaches to neuroscience may not live up to their promise or succeed in advancing the field,” Jonas said.
“Progress requires better experiments, theories, and data analysis approaches,” Kording added.
There are of course some major limitations to the study. Jonas and Kording didn’t apply all techniques neuroscientists use and, the elephant in the room — microprocessors are really different from brains. Still, the findings do suggest that there are limitations to what modern neurosciene can reveal about the brain. The two researchers hope that by trying our hand at reverse-engineering synthetic systems first, we may gain better understanding on how to do the same with the brain.
The full paper “Could a Neuroscientist Understand a Microprocessor?” has been published in the journal PLOS Computational Biology.
Was this helpful?