
Artificial intelligence has the potential to forever change the world because it combines the best of both ‘brains’: the human knack for spotting patterns and making sense of information, and the fantastic raw computing power of machines. A fantastic example of the power of AI in action was demonstrated only last year when Google’s DeepMind division beat world champion and legend Lee Sedol at Go, a game that has 10761 possible moves compared to only 10120 possible in chess. With great power comes great responsibility, however, and many leading scientists and entrepreneurs, among them Stephen Hawking or Elon Musk, have time and time again warned developers that they’re treading on thin ice.
Nick Bostrom, a thin, soft-spoken Swede is probably the biggest A.I. alarmist in the world. His seminal book Superintelligence warns that once artificial intelligence surpasses human intelligence, we might be in for trouble. One famous example Bostrom talks about in his book is the ultimate paper clip manufacturing machine. Bostrom argues that as the machine become smarter and more powerful, it will begin to devise all sorts of clever ways to convert any material into paper clips — that includes humans too.
Recently, the same DeepMind labs ran a couple of social experiments with their neural networks to see how these handle competition and cooperation. This work is very important because it’s foreseeable in the near future that many different kinds of AIs will be forced by circumstances to interact. An AI that manages traffic lights might interfere with another AI that’s tasked with monitoring and controlling air quality, for instance. Which of their goals is more important? Should they compete or cooperate? Nevertheless, the conclusions don’t seem to ease fears concerning AI overlord dominance one bit — on the contrary, they suggest Bostrom’s grotesque paper clip machine isn’t such a far-fetched scenario.
Can you ever have enough apples?
In the first experiment, neural networks were tasked with playing a Gathering game in which the players had to gather as many apples as they could to receive a positive reward. Using multi-agent reinforcement learning, the two agents (Red and Blue) were taught how to behave rationally after thousands and thousands of iterations of the Gathering game. The catch is that each agent was allowed to direct a beam onto the other player which would temporary disable him. This action, however, did not trigger any reward.
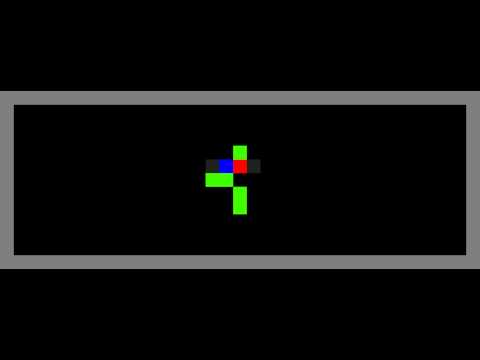
When there were enough apples, the two agents coexisted peacefully. As the number of apples was gradually shrunk down, the agents learned that it was in their best interest to blast their opponents so they got the chance to collect more apples and hence more rewards. In other words, the AI learned greed and aggressiveness — no one thought them that per se. As the Google researchers upped the agents’ complexity (better neural networks), the agents tagged each other more frequently, essentially becoming more aggressive no matter how much the scarcity of the apples was varied.
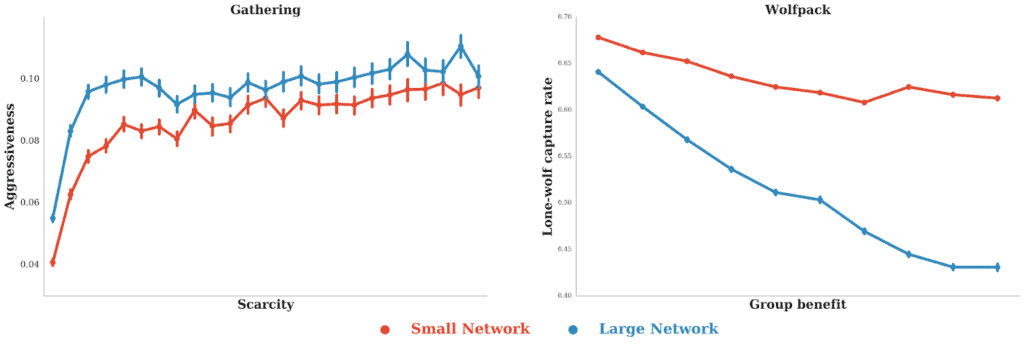
In a second game called Wolfpack, there were three agents this time: two AIs that played as wolves and another that acted as the prey. The wolf would receive a reward if it caught the prey. The same reward was given to both wolves if they were in the vicinity of the carcass no matter who took it down. After many iterations, the AI learned that cooperation was the best course of action in this case instead of being a lone wolf. A greater capacity to implement complex strategies leads to more cooperation between agents or the opposite of the Gathering game.
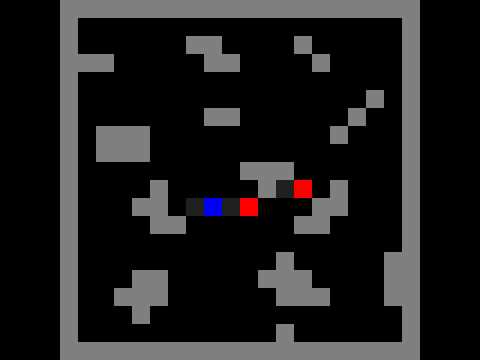
“In summary, we showed that we can apply the modern AI technique of deep multi-agent reinforcement learning to age-old questions in social science such as the mystery of the emergence of cooperation. We can think of the trained AI agents as an approximation to economics’ rational agent model “homo economicus”. Hence, such models give us the unique ability to test policies and interventions into simulated systems of interacting agents – both human and artificial,” the DeepMind team wrote in a blog post.
These results are still preliminary and Google has yet to publish them in a peer-reviewed journal. However, it’s clear that an unabated machine that acts ‘rationally’ will not act in humanity’s best interest just like an individual will not generally act in best interest of his species. There are some big concerns here and a solution isn’t in clear sight. Some have proposed adding a kill switch for AI but that doesn’t seem too reassuring. It means you’re admitting AI could become a threat. Perhaps, it’s inevitable for an A.I. to turn into a giant paper clipping machine if it’s taught to act like a human. So maybe artificial intelligence should be taught to behave less rationally and more kindly. The economics of compassion are harder to pin down, however.
Luckily, AI is still in its infancy. These games are still very simplistic, even Go is, when compared to the complexity of human affairs or the world for that matter. Everyone is approaching this very slowly and carefully — for now.
Was this helpful?