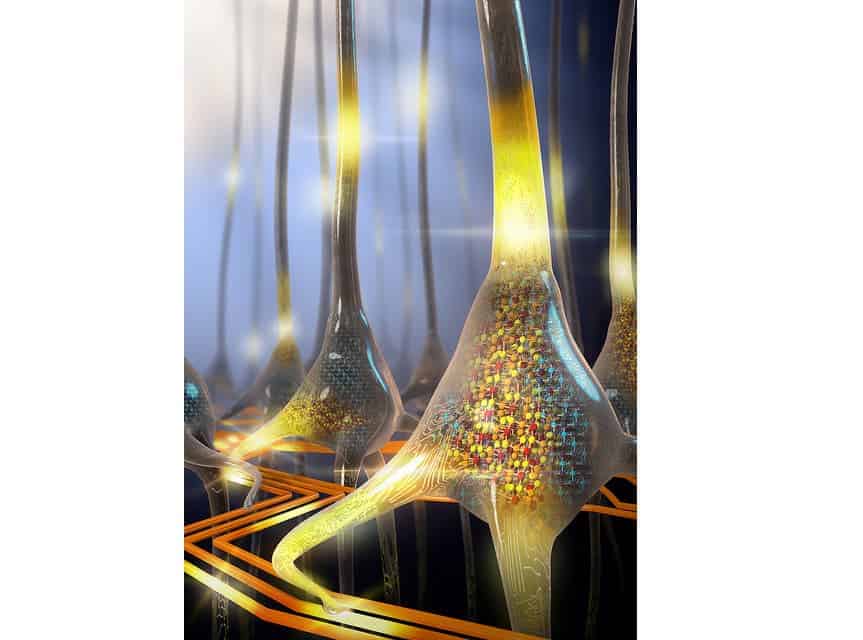
Scientists at IBM-Research Zürich and ETH Zürich claim they’ve made a huge leap in neuromimetic research, which ultimately aims to build a computing machine that closely mimics the human brain. The team imitated large populations of neurons for the very first time and used them to carry out complex computational tasks with remarkable efficiency.
Imitating the most complex biological entity in the universe — the human brain
In a confined space of merely two liters, the human brain is able to perform amazing computational feats requiring only 10 to 20 Watts of power. A supercomputer that would barely mimic the human brain’s computational power would be huge and would require diverting an entire river to keep it cool, were it designed using a classic von-Neumann architecture (the kind your laptop or smartphone uses). With such a great example of biological computing, we’re clearly doing awfully inefficient work right now.
[accordion style=”info”][accordion_item title=”The power of the human brain “]The average human brain has about 100 billion neurons (or nerve cells) and many more neuroglia (or glial cells) which serve to support and protect the neurons (although see the end of this page for more information on glial cells).
Each neuron may be connected to up to 10,000 other neurons, passing signals to each other via as many as 1,000 trillion synaptic connections, equivalent by some estimates to a computer with a 1 trillion bit per second processor. Estimates of the human brain’s memory capacity vary wildly from 1 to 1,000 terabytes (for comparison, the 19 million volumes in the US Library of Congress represents about 10 terabytes of data).[/accordion_item][/accordion]
Mimicking the computing power of the brain, the most complex computational ‘device’ in the universe, is a priority for computer science and artificial intelligence enthusiasts. But we’re just beginning to learn how the brain works and what lies within our deepest recesses – the challenges are numerous. But we’re tackling them one at a time.
It all starts with imitating biological neurons and their synapses. In a biological neuron, a thin lipid-bilayer membrane separates the electrical charge encased in the cell, allowing the membrane potential to be maintained. When the dendrites of the neuron are excited, this membrane potential is altered and the neuron, as a whole, “spikes” or “fires”. Emulating these sort of neural dynamics with conventional CMOS hardware like subthreshold transistor circuits is technically unfeasible.
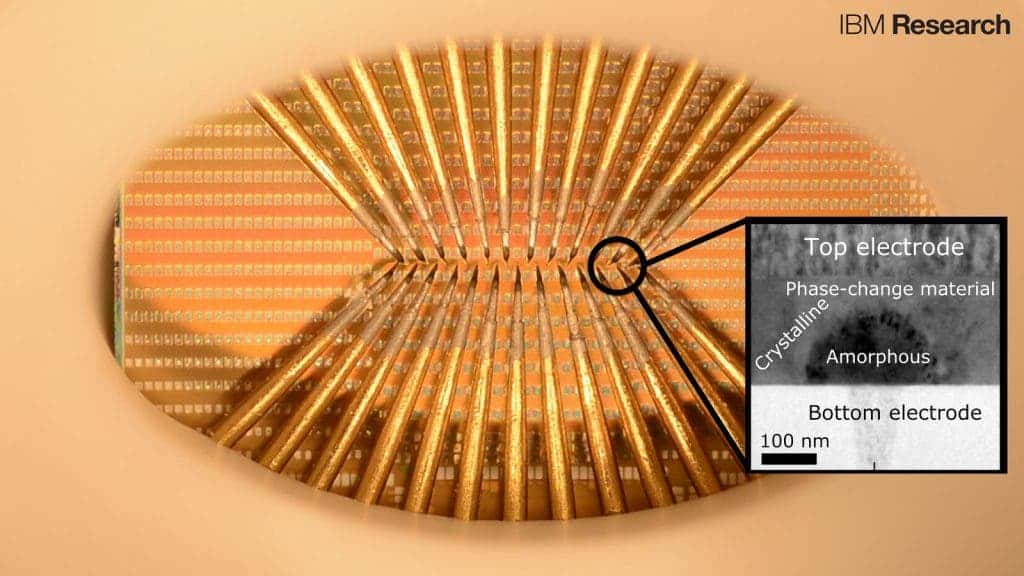
Instead, research nowadays is following the biomimetic route as close as possible. For instance, the researchers from Zürich made a nanoscale electronic phase-change device from a chalcogenide alloy called germanium antimony telluride (Ge2Sb2Te5). This sort of material can quickly and reliably change between purely amorphous and purely crystalline states when subjected to an electrical or light stimulus. The same alloy is used in applications like Bluray disks to store digital information, however, in this particular instance, the electronic neurons are analog, just like the synapses and neurons in a biological brain.
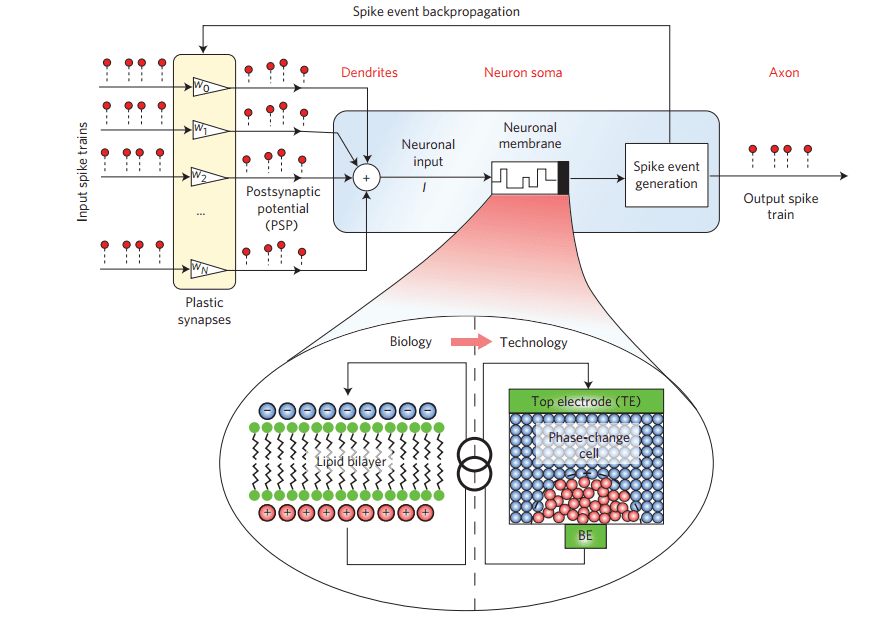
This phase-change material emulates the biological lipid-bilayer membrane and enabled the researchers to devise artificial spiking neurons, consisting of inputs (dendrites), the soma (which comprises the neuronal membrane and the spike-event generation mechanism) and the output (axon). These were assembled in 10×10 arrays. Five such arrays were connected to create a neural population of 500 artificial neurons, more than anyone has come close yet.
“In a von-Neumann architecture, there is a physical separation between the processing unit and memory unit. This leads to significant inefficiency due to the need to shuttle data back and forth between the two units,” said Dr. Abu Sebastian, Research Staff Member Exploratory Memory and Cognitive Technologies, IBM Research – Zurich and co-author of the paper published in Nature Nanotechnology.
“This is particularly severe when the computation is more data-centric as in the case of cognitive computing. In neuromorphic computing, computation and memory are entwined. The neurons are connected to each other, and the strength of these connections, known as synapses, changes constantly as the brain learns. Due to the collocation of memory and processing units, neuromorphic computing could lead to significant power efficiency. Eventually, such neuromorphic computing technologies could be used to design powerful co-processors to function as accelerators for cognitive computing tasks,” Sebastian added for ZME Science.
Prof. David Wright, the head of Nano-Engineering, Science and Technology Group at the University of Exeter, says that just one of these integrate-and-fire phase-change neurons can carry out tasks of surprising computational complexity.
“When applied to social media and search engine data, this leads to some remarkable possibilities, such as predicting the spread of infectious disease, trends in consumer spending and even the
future state of the stock market,” Wright said, who was not involved in the present paper, but is very familiar with the work.
When the GST alloy crystallizes to become conductive, it spikes. What’s amazing and different from previous work, however, is that this firing exhibits an inherently stochastic nature. Scientists use the term stochastic to refer to the randomness or noise biological neurons generate.
“Our main achievements are two fold. First, we have constructed an artificial integrate-and-fire neuron based on phase change materials for the first time. Secondly, we have shown how such a neuron can be used for highly relevant computational tasks such as temporal correlation detection and population coding-based signal representation. For the latter application, we also exploited the inherent stochasticity or randomness of phase change devices. With this we have developed a powerful experimental platform to realize several emerging neural information processing algorithms being developed within the framework of computational neuroscience,” Dr. Sebatian told ZME Science.
The same artificial neurons can sustain billions of switching cycles, signaling they can pass a key reliability test if they’re ever to become useful in the real world, such as embedded in Internet of Things devices or the next generation of parallel computing machines. Most importantly, the energy required for each neuron to operate is awfully low, minuscule even. One neuron update needs only five picojoules of energy to trigger it. In terms of power, it uses less than 120 microwatts or hundreds of thousands of times less than your typical light bulb.
“Populations of stochastic phase-change neurons, combined with other nanoscale computational elements such as artificial synapses, could be a key enabler for the creation of a new generation of extremely dense neuromorphic computing systems,” said Tomas Tuma, a co-author of the paper, in a statement.
The phase-change neurons are still far from capturing the full range of biological neuron traits, but the work is groundbreaking on many levels. Next on the agenda is to make these artificial neurons even more efficient by aggressively scaling down the size of the phase-change devices, Dr. Sebastian said.