DeepMind is one step closer to emulating the human mind. Google engineers claim their artificial neural network can now use store data similarly to how humans access memory.
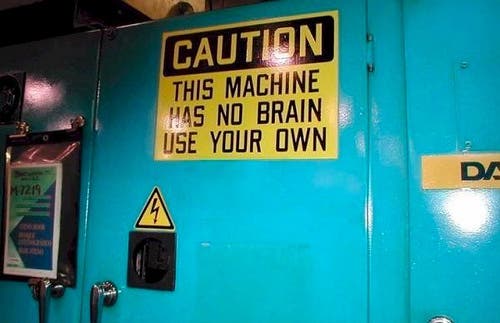
Image credits Pierre-Olivier Carles / Flickr.
The AI developed by Alphabet, Google’s parent company, just received a new and powerful update. By pairing up the neural network’s ability to learn with the huge data stores of conventional computers, the programmers have created the first Differential Neural Computer, or DNC — allowing DeepMind to navigate and learn from the data on its own.
This brings AIs one step closer to working as a human brain, as the neural network simulates the brain’s processing patterns and external data banks supplying vast amounts of information, just like our memory.
“These models… can learn from examples like neural networks, but they can also store complex data like computers,” write DeepMind researchers Alexander Graves and Greg Wayne in a blog post.
Traditional neural networks are really good at learning to do one task — sorting cucumbers, for example. But they all share a drawback in learning to do something new. Aptly called “catastrophic forgetting”, such a network has to erase and re-write everything it knows before being able to learn something else.
Learn like a human, work like a robot
Our brains don’t have this problem because they can store past experience as memories. Your computer doesn’t have this problem either, as it can store data on external banks for future use. So Alphabet paired up the later with a neural network to make it behave like a brain.
The DNC is underpinned by a controller that constantly optimizes the system’s responses, comparing its results with the desired or correct answers. Over time, this allows it to solve tasks more and more accurately while learning how to apply the data it has access to at the same time.
At the heart of the DNC is a controller that constantly optimizes its responses, comparing its results with the desired and correct ones. Over time, it’s able to get more and more accurate, figuring out how to use its memory data banks at the same time. The results are quite impressive.
After feeding the London subway network into the system, it was able to answer questions which require deductive reasoning — which computers are not good at.
For example here’s one question the DNC could answer: “Starting at Bond street, and taking the Central line in a direction one stop, the Circle line in a direction for four stops, and the Jubilee line in a direction for two stops, at what stop do you wind up?”
While that may not seem like much — a simple navigation app can tell you that in a few seconds — what’s groundbreaking here is that the DNC isn’t just executing lines of code — it’s working out the answers on its own, working with the information it has in its memory banks.
The cherry on top, the DeepMind team stated, is that DNCs are able to store learned facts and techniques, and then call upon them when needed. So once it learns how to deal with the London underground, it can very easily handle another transport network, say, the one in New York.
This is still early work, but it’s not hard to see how this could grow into something immensely powerful in the future — just imagine having a Siri that can look at and understand the data on the Internet just like you or me. This could very well prove to be the groundwork for producing AI that’s able to reason independently.
And I, for one, am excited to welcome our future digital overlords.
The team published a paper titled “Hybrid computing using a neural network with dynamic external memory” describing the research in the journal Nature.
Was this helpful?