A new study describes a novel computing component which emulates the way neurons connect in the human brain. This “memristor” changes its electrical resistance depending on how much current has already flowed through it, mimicking the way neurons transmit signals through synapses, the team writes.
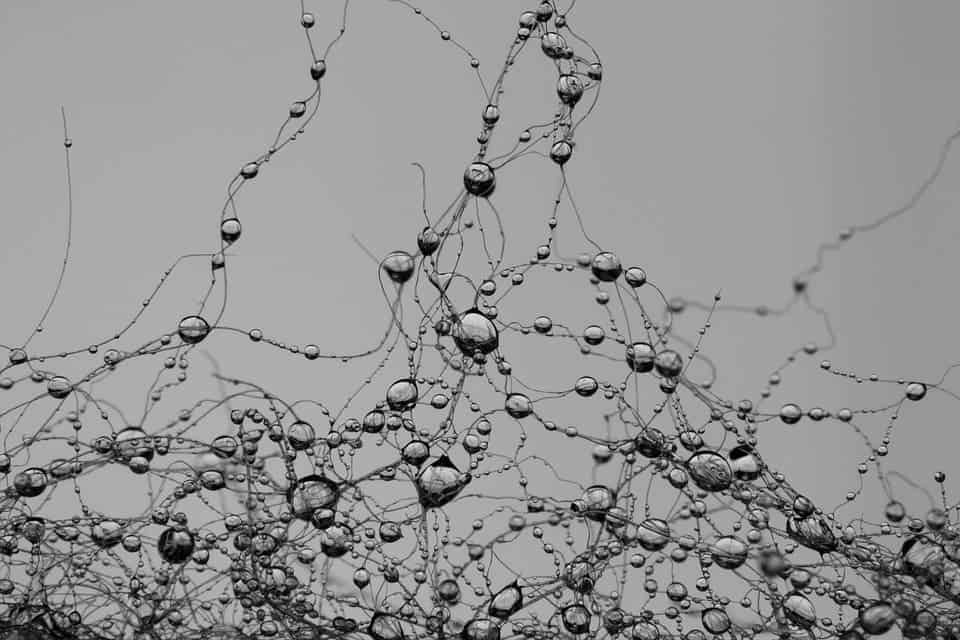
This device could lead to significant advancements in brain-like computers, capable of handling perceptual and learning tasks much better that traditional computers while being much more energy efficient.
“In the past, people have used devices like transistors and capacitors to simulate synaptic dynamics, which can work, but those devices have very little resemblance to real biological systems,” said study leader and professor of electrical and computer engineering at the University of Massachusetts Amherst Joshua Yang.
The human brain has somewhere between 86 to 100 billion neurons which connect in up to 1,000 trillion (that’s a one followed by 15 zeros) synapses — making your brain an estimated 1 trillion bit per second processor. Needless to say, computer scientists are dying to build something with even a fraction of this processing power, and a computer that mimics the brain’s structure — and thus its computing power and efficiency — would be ideal.
Building a brain
When an electrical signal hits a synapse in your brain it prompts calcium ions to flood into it, triggering the release of neurotransmitters. This is what actually transmits the information over the synapse, causing an impulse to form in the other neuron and so on. The “diffusive memristor” described in the paper is made up of silver nanoparticle clusters embedded in a silicon oxynitride film that is embedded between two electrodes.
The film is an insulator but applying a voltage through the device and the clusters start to breaks apart through a combination of electrical forces and heat. The nanoparticles diffuse through the film to form a conductive filament, allowing current to flow from one electrode to the other. Cut the voltage, the temperature drops, and the clusters re-form — similar to how calcium ions behave in a synapse.
“With the synaptic dynamics provided by our device, we can emulate the synapse in a more natural way, more direct way and with more fidelity,” he told Live Science.
The device can thus mimic short-term plasticity in neurons, the researchers said. Trains of low-voltage, high-frequency pulses will gradually increase the device’s conductivity until a current can pass through. But, if the pulses continue, the conductivity will eventually decrease.
The team also combined their diffusion memristor with a drift memristor, which relies on electrical fields and is optimized for memory applications. This allowed them to demonstrate a form of long-term plasticity called spike-timing-dependent plasticity (STDP), adjusting connection strength between neurons based on the timing of impulses. Drift memristors have previously used to approximate calcium dynamics. But, because they’re based on physical processes very different from the ones our brains employ, they have limited fidelity and variety in what functions they can simulate.
“You don’t just simulate one type of synaptic function, but [also] other important features and actually get multiple synaptic functions together,” Yang said.
“The diffusion memristor is helping the drift-type memristor behave similarly to a real synapse. Combining the two leads us to a natural demonstration of STDP, which is a very important long-term plasticity learning rule.”
Reproducing synaptic plasticity is essential to creating a brain-like computer. And we should do our best to create one, Yang said.
“The human brain is still the most efficient computer ever built,” he added.
The team uses fabrication processes similar to those being developed by computer memory companies to scale up memristor production. Silver doesn’t lend well to all these methods, however, copper nanoparticles could be used instead, Yang said. He added that the approach is definitely scalable and single units systems should be comparable to biological synapses in size. But he added that in multiunit systems, the devices will likely need to be bigger due to practical considerations involved in making a larger system work.
The full paper “Memristors with diffusive dynamics as synaptic emulators for neuromorphic computing” has been published in the journal Nature Materials.
Was this helpful?