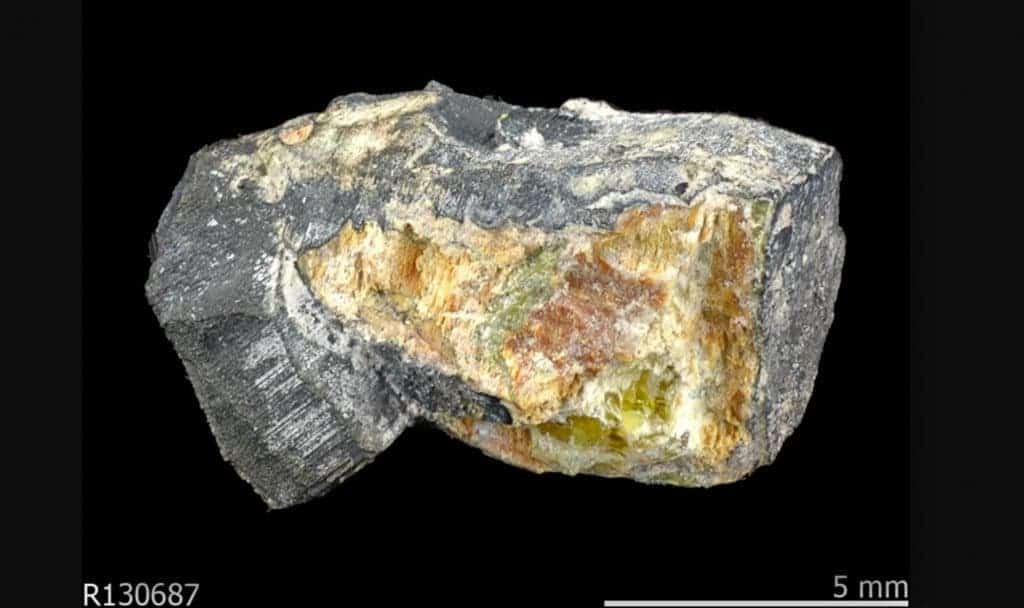
The same approach that Facebook uses to graph networks or scientists employ to map the spread of diseases was applied to predict new minerals species and deposits. It’s the first time network theory and big data — a buzz word that describes the uses of huge data sets to reveal patterns and make predictions otherwise difficult to obtain — have been employed to find new minerals.
High-tech geology
We know of about 5,200 mineral species, each of which has a unique combination of chemical composition and atomic structure. There are millions of mineral samples housed in museums, warehouses, universities or private collections, many of which have been neatly described and cataloged. For instance, it’s standard practice for a mineral sample to be tagged with information like the location it was recovered from, the level or occurrence, the age of the deposit or the mineral’s growth rate.
When you combine this information — not on one deposit but a myriad — with data on the surrounding geography, the geological setting, and coexisting minerals, it’s possible to fill in the blanks and infer the existence of not only deposits but also new mineral species.
It’s only recently that the technology has enabled scientists to use such a ‘big data’ approach.
“The quest for new mineral deposits is incessant, but until recently mineral discovery has been more a matter of luck than scientific prediction,” said Dr. Shaunna Morrison of the Deep Carbon Observatory in a statement. “All that may change thanks to big data.”
Just like data scientists use complex data sets to understand social media connections, city traffic or even metabolic pathways, so can the same method apply to mineralogy and petrology. There are really few fields of science where big data can’t help make a breakthrough.
Bearing this in mind, Morrison and colleagues were able to visualize data from multiple variables on thousands of mineral samples sourced from hundreds of thousands of locations around the world. And it was all represented within a single graph which reveals patterns of occurrence and distribution otherwise extremely difficult if not impossible to infer.
From here on, it’s only a matter of filling the gaps of a list of minerals. Moreover, the analysis also tells us where we can dig to find new deposits. Robert Hazen at the Carnegie Institution for Science discusses mineral evolution, mineral ecology, and mineral network analysis in this hour-long lecture embedded below.
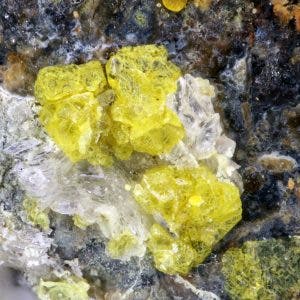
The hunt for new minerals is on
Already, this approach enabled the researchers to predict the existence of 145 missing carbon-bearing minerals and where to find them. To accelerate their discovery, the Deep Carbon Observatory launched the Carbon Mineral Challenge to inspire professional and amateur mineralogists alike to hunt down minerals from this shortlist. Already, ten have been found. Among them is ewingite, the most structurally complex known mineral on Earth. Many more minerals await discovery, though.
“We have used the same kinds of techniques to predict that at least 1,500 minerals of all kinds are ‘missing,’ to predict what some of them are, and where to find them,” Dr. Hazen says.
Analyses of complex mineral datasets also help to untangle some of the intricate relationships between geology and biology, leading to new insights into the co-evolution of the geosphere and biosphere. For instance, mineral networks of igneous rocks can help retrace ‘Bowen’s reaction series’, which tells us how various characteristic minerals form once magma cools. The analysis was so precise that the predicted sequence of minerals matched reality precisely. In the future, mineral networks coupled with data on biomarker molecules can reveal insights on how cells and minerals interact.
Mineral networks can also serve as a powerful learning tool in academia for mineralogy and petrology, helping students visualize rocks, minerals and their relationships.
And of course, the industry could make billions by mining new deposits. Some of the yet to be identified minerals could have remarkable properties that might enable novel products on the market. Really, there are countless applications of big data on minerals and the implications could be far reaching.
“Minerals provide the basis for all our material wealth,” Morisson concluded, “not just precious gold and brilliant gemstones, but in the brick and steel of every home and office, in cars and planes, in bottles and cans, and in every high-tech gadget from laptops to iPhones.”
“Minerals form the soils in which we grow our crops, they provide the gravel with which we pave our roads, and they filter the water we drink.”
“This new tool for understanding minerals represents an important advance in a scientific field of vital interest.”
Findings appeared in the journal American Mineralogist.
Was this helpful?