
It’s in the interest of any government to have timely and accurate demographic statistics concerning its citizens. Conventional, door-to-door surveying can take a very long time to process, as well as cost a lot of money. The yearly American Community Survey (ACS), for instance, costs taxpayers $250 million. A group of researchers might have discovered a better alternative, however. Using deep learning techniques, the scientists tapped into the enormous Google Street View database to measure statistics like race, gender, education, occupation, unemployment, and other demographic factors by simply analyzing what automobiles people drive.
Some 50 million images of street scenes from regions across 200 cities were fed into an AI that then estimated socioeconomic characteristics by using vehicles as a proxy. Convolutional Neural Networks (CNN), a computer vision technique, was then employed to determine the make, model, and year of all motor vehicles encountered in particular neighborhoods — some 22 million automobiles in total, comprising 8% of all automobiles in the nation. The same task would have taken a human expert 15 years to complete.
“Differences between cars can be imperceptible to an untrained person; for instance, some car models can have subtle changes in tail lights (e.g., 2007 Honda Accord vs. 2008 Honda Accord) or grilles (e.g., 2001 Ford F-150 Supercrew LL vs. 2011 Ford F-150 Supercrew SVT). Nevertheless, our system is able to classify automobiles into one of 2,657 categories, taking 0.20.2 s per vehicle image to do so,” the authors wrote.
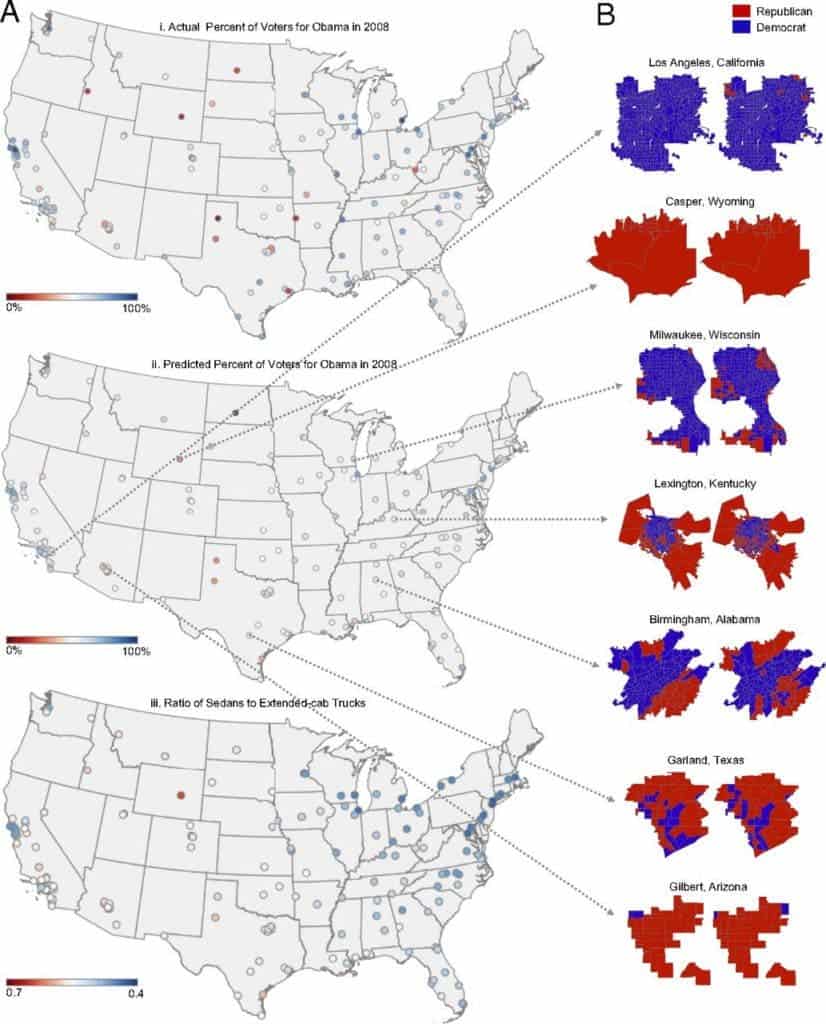
For each geographical region that the researchers examined (city, zip code, or precinct), the number of vehicles of each make and model was calculated. A smaller batch comprising 35 of the 200 cities was then used as a training dataset for the machine which was taught to estimate race and education levels based on ACS and presidential election voting data.
“This simple linear model is sufficient to identify positive and negative associations between the presence of specific vehicles (such as Hondas) and particular demographics (i.e., the percentage of Asians) or voter preferences (i.e., Democrat).”
The results confirm previous socio-economic findings. For instance, people of Asian descent are more likely to drive cars from Asian manufacturers, particularly Hondas and Toyotas. Cars manufactured by Chrysler, Buick, and Oldsmobile are positively associated with African American neighborhoods, which is again consistent with existing research. On the other hand, pickup trucks, Volkswagens, and Aston Martins are indicative of mostly Caucasian neighborhoods.
One of the most interesting results was that in Democratic precincts the vehicles of choice were sedans, whereas Republican precincts were most strongly associated with extended-cab pickup trucks. The researchers found that if there were more sedans than pickup trucks, there’s an 88% chance the city in question voted Democrat, and if there are more pickup trucks, it’s 82% likely that it voted Republican. The results were verified with actual ACS data, city-by-city, across all tested cities.
“These results illustrate the ability of our machine-learning algorithm to accurately estimate both demographic statistics and voter preferences using a large database of Google Street View images. They also suggest that our demographic estimates are accurate at higher spatial resolutions than those available for yearly ACS data. Using our approach, zip code- or precinct-level survey data collected for a few cities can be used to automatically provide up-to-date demographic information for many American cities.”
The American Community Survey (ACS) performed by the US Census Bureau is not only labor intensive and costly but also sometimes fails to encompass the full scope of the nation’s demographics. That’s partly because ACS only surveys cities and counties with a population of 65,000 or more, while smaller regions are interrogated far less frequently. In contrast, a computational approach can potentially analyze “demographic trends in great detail, in real time, and at a fraction of the cost,” wrote the study’s authors in the Proceedings of the National Academy of Sciences.
In the future, as more and more Americans use driverless cars littered with onboard cameras, the wealth of data will help us obtain even more accurate information at a more granular level than ever more. For instance, every single day, Tesla cars around the country take as many images as were utilized in this study — and there were quite a lot.
Such work highlights a recent trend in social sciences where computational methods are used to tackle complex problems. Previously, for instance, scientists predicted unemployment rates from Twitter and used mobile phone metadata to assess poverty rates in Rwanda.