Could AI help tutor individuals? New research from the Defense Advanced Research Projects Agency (DARPA) seems to suggest that yes — and extremely well, according to Delta Academy.
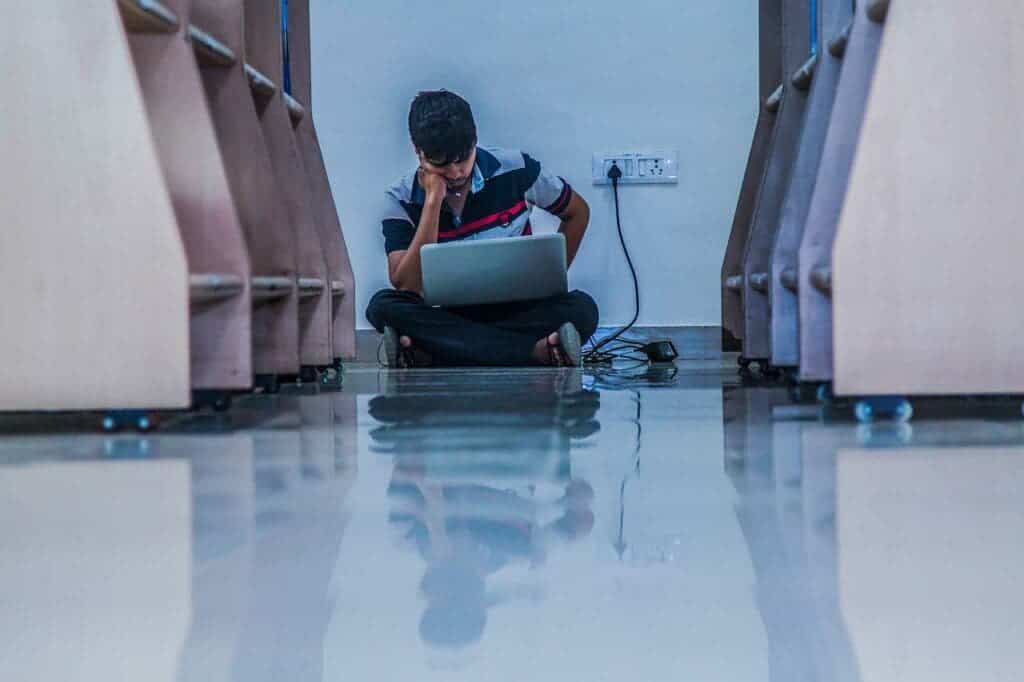
It would be hard to believe that professional juniors, fresh off of the school bench, could outperform seasoned vets with years of experience. And yet, that is exactly what DARPA achieved using an artificial intelligence (AI) training algorithm called the Digital Tutor for Naval IT Training. This system retains the sheer efficiency of one-to-one tutoring, the most effective training method we’ve been able to find in all of history, while doing away with its most glaring limitation: it is not scalable; one tutor can only handle one pupil at a time.
The findings point to the role AI can have in training individuals of all ages. Such an approach would allow much greater numbers of pupils to access high-quality training in a cost-efficient manner, while also producing outstanding educational outcomes. In a round of DARPA testing, new recruits that spent 16 weeks training with the AI significantly outscored traditionally-trained recruits as well as seasoned personnel (with 5 years’ worth of experience) by a significant margin.
Computer classes
“Digital Tutor teams attempted a total of 140 problems and successfully solved 74% of them (104 problems), with an average score of 3.78 (1.91). Fleet teams attempted 100 problems and successfully solved 52% of them (52 problems), with an average score of 2.00 (2.26). ITTC [standard-training] teams attempted 87 problems and successfully solved 38% of them (33 problems), with an average score of 1.41 (2.09).”
DARPA’s stated goal was to “capture in computer technology the capabilities of individuals who were recognized experts in a specific area and proficient in one-on-one tutoring”. But this is a prime example of “easier said than done”.
The construction process for the Digital Tutor was a huge undertaking and relied on a great deal of access to expert knowledge. These experts were asked to transmit the knowledge and skills required in their profession in painstaking detail while drawing on existing reference materials, courses, and direct interviews. Almost half of the project’s total funding was spent identifying these experts and recording the data.
Based on this immense body of knowledge, the experts then designed a 16-week, human-led course, which they taught. All conversations held between the human tutors and their students during this course were recorded. These recordings helped further shape the Digital Tutor. Based on the interactions, additional features that the students might need were included in the system, the final ontology was developed, and the algorithms behind the AI were further refined.
Unlike other technologies that include machines in learning today, which rely on computers assisting human teachers, the Digital Tutor handled the training itself, with human mentors providing assistance. The system is ‘problem-based’, the agency explains, so that it can better match the difficulty of the material it presents with the skill of the pupil.
It first presents explanatory material to the user and then supplies a series of problems to be solved using that material. Throughout that process, it produces a model of each individual learner. Through the use of this approximate knowledge model, the system can ensure that each learner has understood the concepts and issues shown. The lessons and material presented are further adapted based on this model. For example, faster learners are given extension exercises, while those that are struggling are walked through the parts they are finding difficult.
During the DARPA tests, all learners were given the same training time inside the Digital Tutor over a 16-week period. The system handled all the processes, providing the appropriate resources for each learner to work with during that time. This seems to have been a winning approach.
“Observers noted that the Digital Tutor established the same kind of concentration, involvement, and flow that is characteristic of interactive computer game playing,” an analysis of the system’s performance reports.
Due to the nature of this research, we don’t have much detail about how the backend of the system works. What we do know is that it is constructed out of three core components: an inference engine, an instruction engine, and a conversation module. The first one observes how the learner is trying to solve the problem they are presented with, the second one decides which problem to display next, and the third delivers the problem through a text-based interface. This conversation module allows questions and responses to be transmitted in natural language, although it does not support free-form language.
The results of students trained using this Digital Tutor showcase just how powerful a technology it can be. That being said, we are probably still quite a way away from sending our kids off to class to study under their digital teachers. One of the most significant limitations right now is that such a system can only work in domains where knowledge can be defined clearly — think math over literature. Until we figure out a way to make the system more adaptable, so that it can tackle the murkier process of general learning, then, human teachers can rest assured: AI is not coming to take their jobs.