Security analysts rely on all sorts of automated software that spots suspicious activity. Even so, an analyst has to churn through even thousands of false positives on a daily basis, which makes it easy to miss a cyber attack. Coming to their rescue is MIT, which reports an artificial intelligence ‘tutored’ by the best human experts which can identify 85 percent of incoming attacks. Most importantly, it’s not confined to a certain set of attack patterns and learns to adapt with each new attack.
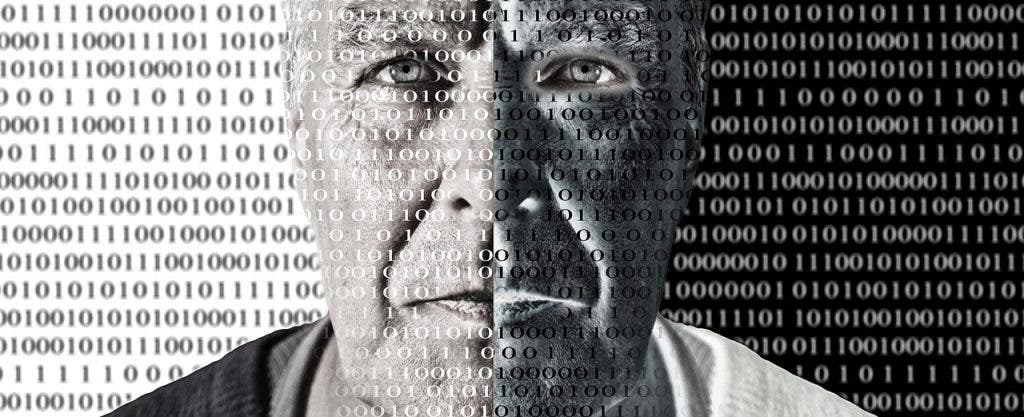
The artificial intelligence developed at MIT’s Computer Science and Artificial Intelligence Laboratory(CSAIL) is called AI2 . The name, the researchers say, signifies a merger between artificial intelligence and human analyst intuition — the best of both worlds.
AI2 has a wealth of data and patterns fed into its ‘brain’ which it uses to detect suspicious activity. It then reports this activity to a human analyst who can then judge if there’s an actual attack. Tests were made on an e-commerce platform which has 40 million log lines each day. In total, AI2 churned through 3.9 billion lines of server activity and detected 85 percent of the actual threats, while also reducing the number of false positives by a factor of 5.
“You can think about the system as a virtual analyst,” says CSAIL research scientist Kalyan Veeramachaneni, who developed AI2 with Ignacio Arnaldo, a chief data scientist at PatternEx and a former CSAIL postdoc. “It continuously generates new models that it can refine in as little as a few hours, meaning it can improve its detection rates significantly and rapidly.”
The A.I. uses three different unsupervised-learning methods, the first being feedback given by experts. Initially, the machine compiles a list of threats for the expert to review, and each feedback will improve the machine’s ability to find the needle in the haystack. For instance, AI2 might report a list of 200 potential attacks to the security analyst, but only 30 or 40 after a couple days of training, MIT News reports.
“This paper brings together the strengths of analyst intuition and machine learning, and ultimately drives down both false positives and false negatives,” says Nitesh Chawla, the Frank M. Freimann Professor of Computer Science at the University of Notre Dame. “This research has the potential to become a line of defense against attacks such as fraud, service abuse and account takeover, which are major challenges faced by consumer-facing systems.”
“The more attacks the system detects, the more analyst feedback it receives, which, in turn, improves the accuracy of future predictions,” Veeramachaneni says. “That human-machine interaction creates a beautiful, cascading effect.”
As cyber attacks become increasingly sophisticated, it’s good to hear about such efforts where man and machine can combine their strengths.