Human social behavior is strikingly governed by laws that describe how the masses congregate or interact one another. Yet, despite the empirical evidence, scientists know very little what stems these mass behaviors. An individual is highly unpredictable, but masses are not (in this respect, Isaac Asimov’s Foundation takes it to the extreme with his fictional psychohistory). Now, some of these laws that predict the probability that one person will befriend another or the size of the cities they live in have been explained by mathematicians at the Harvard-Smithsonian Centre for Astrophysics in Cambridge. According to the researchers, these so-called urban behavioral laws are mathematically equivalent to the way galaxies grow in space.
Cities and galaxies have much in common
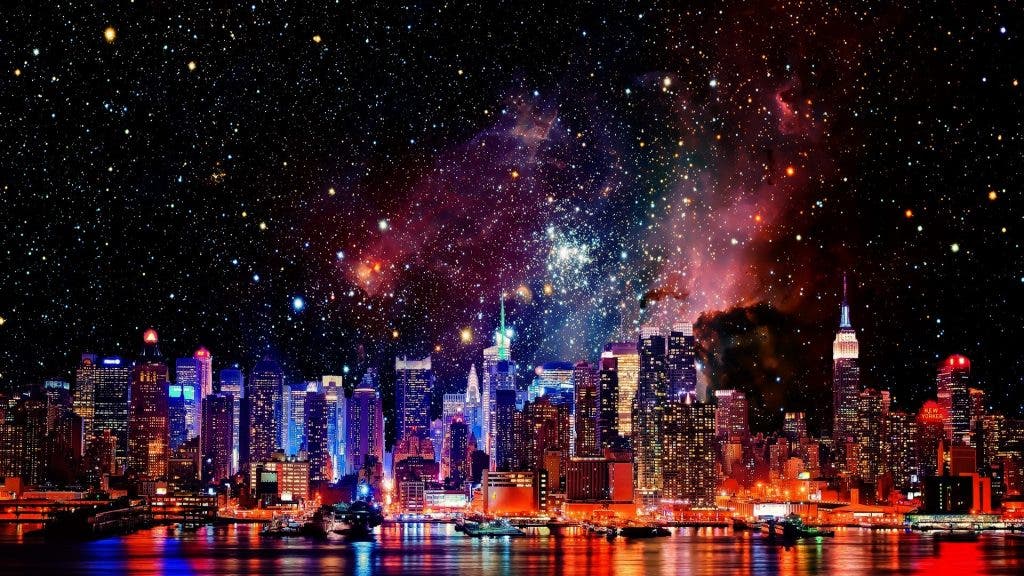
The spatial and density distribution of cities throughout the world is freakishly predicted by an empirical law called Zipf’s law. American linguist George Kingsley Zipf noticed that given some corpus of natural language utterances, the frequency of any word is inversely proportional to its rank in the frequency table. Thus the most frequent word will occur approximately twice as often as the second most frequent word, three times as often as the third most frequent word, etc. For example, in the Brown Corpus of American English text, the word “the” is the most frequently occurring word, and by itself accounts for nearly 7% of all word occurrences (69,971 out of slightly over 1 million). True to Zipf’s Law, the second-place word “of” accounts for slightly over 3.5% of words (36,411 occurrences), followed by “and” (28,852). Only 135 vocabulary items are needed to account for half the Brown Corpus. The same law applies to cities: If cities are listed according to size, then the rank of a city is inversely proportional to the number of people who live in it. Say the biggest city in a country is 8 million people large, then the second biggest will house 8 million divided by two, the third biggest 8 million divided by four and so on.
This relationship is known as the scaling law, exemplified by another urban case: the probability that one person will be friends with another turns out to be inversely proportional to the number of people who live closer to the first person than the second.
Henry Lin and Abraham Loeb at the Harvard-Smithsonian Centre for Astrophysics in Cambridge made a mathematical model of the way human population density varies across a flat Euclidean plane, thus ignoring the curvature of the Earth. Their approach was very similar to how cosmologists consider the evolution of galaxies: first they consider the matter density in the early Universe; then follows the mathematical structure of any variation in density; finally, they explain how this density can change over time as more matter is added or taken away from specific regions.
Lin and Loeb tested the model against publicly available data, and found “the results are in good agreement with the theoretical prediction across a broad range of spatial scales, from a few km to ∼ 10^3 km.” Using the model, the researchers calculated the number of cities past a certain population threshold and found their quantity has a logarithmic slope equal to -1. “This statement is equivalent to Zipf’s law: the rank of a city is inversely proportional to its size,” point out Lin and Loeb. They also computed the average number of friends a person might have within a given region. And once again their model comes up with the inverse-rank friendship law that urban sociologists are already familiar with.
Moreover, initial conditions seems to matter very little as the model always comes up with the same rules. It’s easy to think this is just some mathematical show and tell, but the findings are quite important since the same model could be used to follow other factors that are related to population density, such as the spread of disease.
“Just as the development of models for non-linear structure formation in the universe led to a wealth of theoretical and observational work in cosmology, future work here could include the calculation of new observables such as the bias factor for the spread of epidemics,” they conclude.
Via Technological Review