UK researchers have improved upon a pre-existing AI, allowing it to teach itself how to play three difficult board games: chess, shogi, and Go.
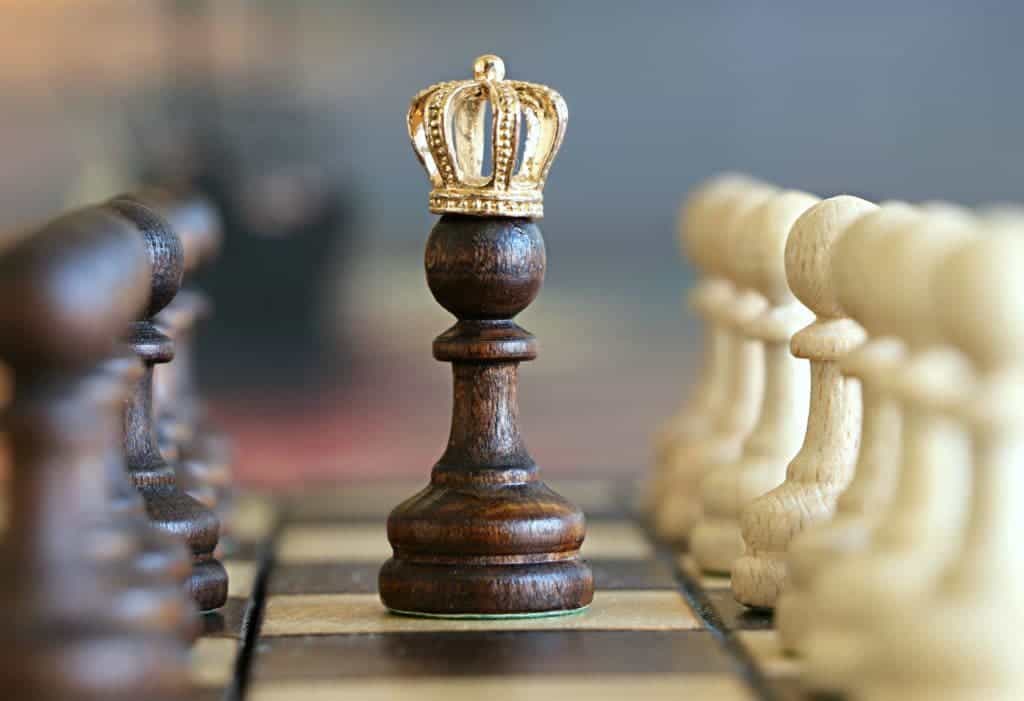
Can’t find a worthy opponent to face in your favorite board game? Fret not! Researchers at the DeepMind group and University College, both in the UK, have created an AI system capable of teaching itself (and mastering) three such games. In a new paper, the group describes the AI and why they believe it represents an important step forward for the development of artificial intelligence.
Let’s play a game
“This work has, in effect, closed a multi-decade chapter in AI research,” Murray Campbell, a member of the team that designed IBM’s Deep Blue, writes in a commentary accompanying the study.
“AI researchers need to look to a new generation of games to provide the next set of challenges.”
Nothing puts the huge strides AI has made over the years into perspective quite like having one beat you at a game. Over two decades ago, an AI known as Deep Blue managed such a feat in a chess game against world champion Gary Kasparov in 1997. Since then, the machines have also managed victories in shogi and Go (think of them as Japanese and Chinese versions of chess).
While impressive, such achievements also showcased the shortcomings of these computer opponents. These programs were good at their respective game — but only at playing that one game. In the new paper, researchers showcase an AI that can learn and master multiple games on its own.
Christened AlphaZero, this AI is based closely on the AlphaGo Zero software and uses a similar reinforcement learning system. Much like a human would, it learns through trial and error by repeatedly playing a game and looking at the results of its actions. All we have to do is explain the basic rules of the game, and then the computer starts playing — against itself. Repeated matches let AlphaZero see which moves help bring about a win, and which simply don’t work.
Over time, all this experience lets the AI become quite adept at the game. AlphaZero has shown that given enough time to practice, it can come to defeat both human adversaries and other dedicated board game AIs — which is no small feat. The system also uses a search method known as the Monte Carlo tree search. Combining the two technologies allows the system to teach itself how to get better at playing a game.

Image credits DeepMind Technologies Ltd
It certainly did help that the team ran the AI on a very beefy platform — the rig employed 5000 tensor processing units, which is on a par with the capabilities of large supercomputers.
Still, AlphaZero can handle any game that provides all the information that’s relevant to decision-making. The new generation of games to which Campbell alluded earlier do not fit into this category. In games such as poker, for example, players can hold their cards close to their chests (and thus obfuscate relevant information). Other examples include many multiplayer games, such as StarCraft II or Dota. However, it likely won’t be long until AlphaZero can tackle such games as well.
“Those multiplayer games are harder than Go, but not that much higher,” Campbell tells IEEE Spectrum. “A group has already beaten the best players at Dota 2, though it was a restricted version of the game; Starcraft may be a little harder. I think both games are within 2 to 3 years of solution.”
The paper “Mastering board games” has been published in the journal Science.
Was this helpful?