A new approach might change how we study our planet’s climate.

When it comes to information, the world has plenty of it. Data sets are increasing rapidly in almost all fields imaginable, with researchers having access to a wealth of data they couldn’t have even dreamed of a few decades ago.
The question is no longer whether you have access to data, but rather how you process and interpret it. This is the so-called big data problem.
Humans and Data
Big data has offered a number of much-needed innovations in modern society. It helped improve health care (by providing personalized medicine and prescriptive analytics, clinical risk intervention and predictive analytics), infrastructure design (by helping planners understand how many cars are on the road at a given time), education (by helping universities design education programs tailored to fit the demand), and many more. But with regards to climate, big data has been less impactful.
There are a few reasons why this has been the case, amongst them the sheer diversity of the available data as well as the inhomogeneities in data availability (i.e. we have more data from densely populated cities than isolated areas). But without a doubt, Georgia researchers say, methodology is also to blame. Too often, scientists employ methods that offer simplistic “yes or no” answers instead of painting an accurate picture. This is counterproductive and needs to change.
“It’s not that simple in climate,” said Annalisa Bracco, a professor in Georgia Tech’s School of Earth and Atmospheric Sciences. “Even weak connections between very different regions on the globe may result from an underlying physical phenomenon. Imposing thresholds and throwing out weak connections would halt everything. Instead, a climate scientist’s expertise is the key step to finding commonalities across very different data sets or fields to explore how robust they are.”
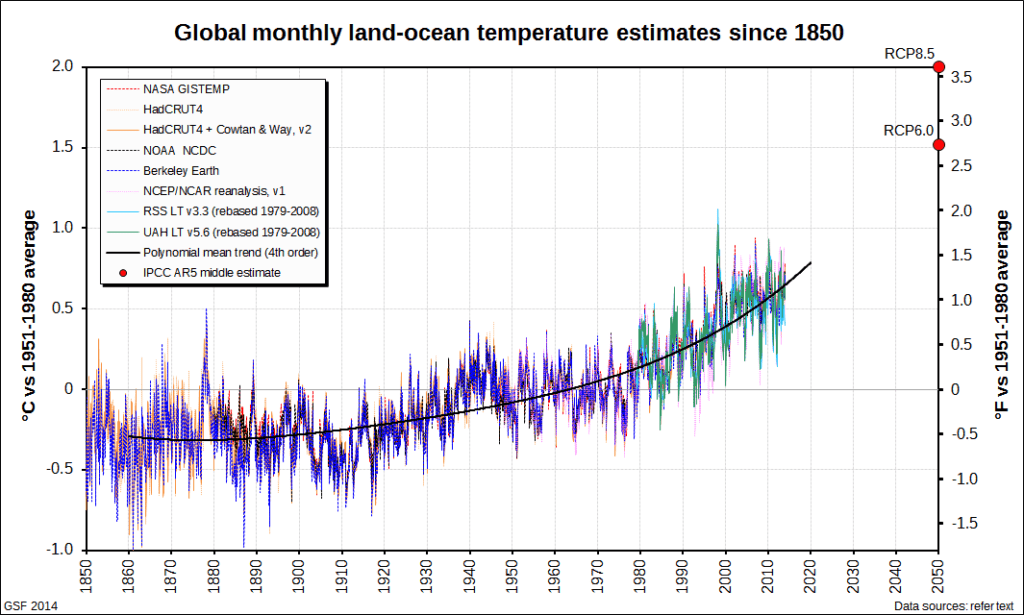
She and her colleagues wanted to develop a data analysis method that relies more on the data itself and less on interpreters’ viewpoints and skills, so they developed a way to mine data from climate data sets that is more self-contained than traditional tools. They claim that this method creates results that are more robust and transparent. In other words, the data will be mined in such a way that two different people analyzing it will reach the same conclusion — something which is not always the case currently.
To make things even better, the methodology is open source and currently available to scientists (or passionate amateurs) around the world.
Another advantage of this approach is that it allows researchers to integrate several data types more easily. Climate is a complex, multifaceted issue, and it’s often hard to make sense of every bit of information from the field.
Furthermore, researchers can also operate the data without having an extensive knowledge of modeling and hardcore coding — something which was becoming more and more of a challenge as most climate scientists don’t have a background in coding.
“There are so many factors — cloud data, aerosols and wind fields, for example — that interact to generate climate and drive climate change,” said Athanasios Nenes, another College of Sciences climate professor on the project. “Depending on the model aspect you focus on, they can reproduce climate features effectively — or not at all. Sometimes it is very hard to tell if one model is really better than another or if it predicts climate for the right reasons.”
It remains to be seen whether or not this approach will enjoy success, but it certainly has the opportunity to foster a newer, simpler, and systematic approach to studying climate.
“Climate science is a ‘data-heavy’ discipline with many intellectually interesting questions that can benefit from computational modeling and prediction,” said Dovrolis, a professor in the School of Computer Science, “Cross-disciplinary collaborations are challenging at first — every discipline has its own language, preferred approach and research culture — but they can be quite rewarding at the end.”
The paper, “Advancing climate science with knowledge-discovery through data mining,” has been published in Climate and Atmospheric Science, a Nature journal.
Was this helpful?