Researchers from Columbia University’s (CU) School of Engineering have developed a robot that can learn what it is by itself.
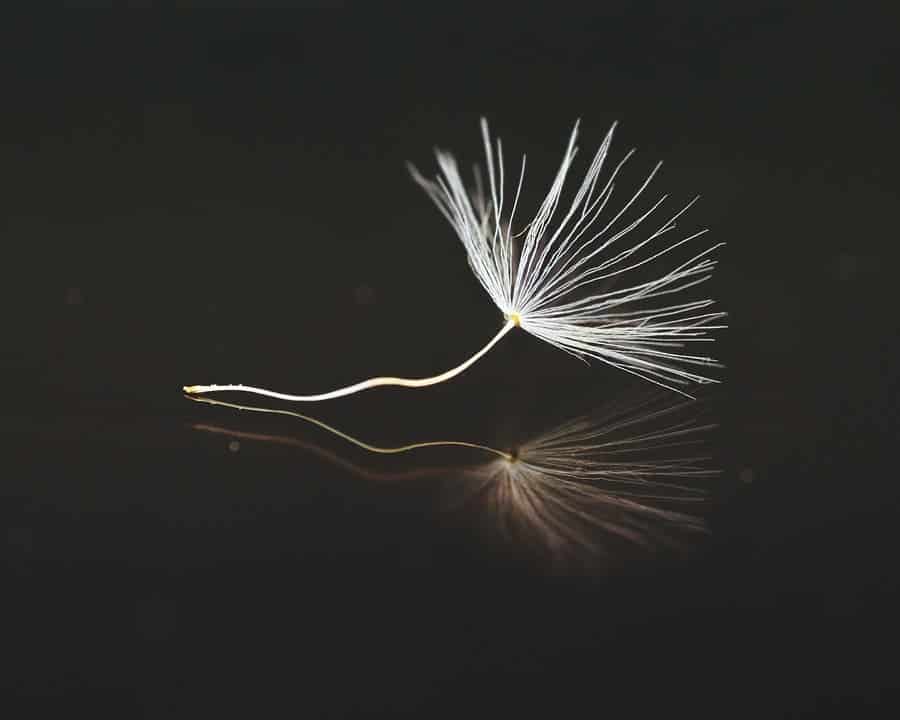
To the best of our knowledge, we’re the only creatures that can imagine ourselves in abstract situations. We can project an image of ourselves in the future, for example, walking down the street or taking an exam. We can also look back on past experiences and reflect upon what went wrong (or right) in a certain scenario — and it’s likely other animals can do this as well. This self-image is fluid, meaning we can adapt and change how we see ourselves over time.
Robots, however, can’t do this yet. We don’t know how to program them to learn from past experience or imagine themselves the way we do. Hod Lipson, a professor of mechanical engineering at CU and director of the Creative Machines lab, and his team are working to change this.
Mirror, mirror on the wall
“If we want robots to become independent, to adapt quickly to scenarios unforeseen by their creators, then it’s essential that they learn to simulate themselves,” says Lipson.
Robots today operate based on models and simulations (of themselves, other objects, or various tasks) provided by a human operator. Lipson, along with Ph.D. student Robert Kwiatkowski, worked to develop a robot that can provide such models for itself, without being offered prior knowledge of physics, its own geometry, or its motor dynamics.
The duo worked with an articulated robotic arm with four degrees of freedom — meaning it could move up-down and left to right, but not backward or forwards in space. The bot was allowed an initial period of “babbling”. It moved randomly and calculated approximatively one thousand trajectories that it could perform, each comprised of one hundred different points.
This data was processed through deep learning software to create a self-model of the robot (this process took about a day). The first such models were pretty bad; they were very inaccurate and didn’t provide a good reflection of the robot’s shape, the position of its joints, and the dimensions were out of scale. A further 35 hours spent refining the model made it more consistent with the robot’s physical shape (with an accuracy of within four centimeters, the team notes).
The next step was to test whether this self-model would actually help (or if it would impede) the robot’s functionality. The team pitted it against a pick-and-place test, working in a closed loop system. This system allowed the bot to determine its starting position during each step of the test based solely on the internal self-model and the trajectories it moved along during the previous step. Overall, it worked surprisingly well — the robotic arm was able to grasp objects in specific locations and deposit them into a receptacle with a 100% success rate.
Switching to an open-loop system — where motions were dictated entirely by the internal self-model without external feedback — the robot managed a (still decent) 44% success rate in the same task. I say still decent because not even us humans normally handle tasks in open-loop systems.
“That’s like trying to pick up a glass of water with your eyes closed, a process difficult even for humans,” says Kwiatkowski, who was the paper’s lead author.
Besides the pick-and-place task, the two also had the robot handle tasks such as writing text with a marker. The paper doesn’t say how well it did, noting that the “task was used for qualitative assessment only”.
Adapt, change, overcome
Another very exciting possibility for such self-models is that they could allow robots to detect damage to themselves. To test how well they could work, the team 3D-printed a deformed part and installed it on the robotic arm (this was meant to simulate damage incurred during operation). The robot was able to detect the change and re-write its model accordingly. This new model allowed the robot to perform the pick-and-place task “with little loss of performance”, the authors report.
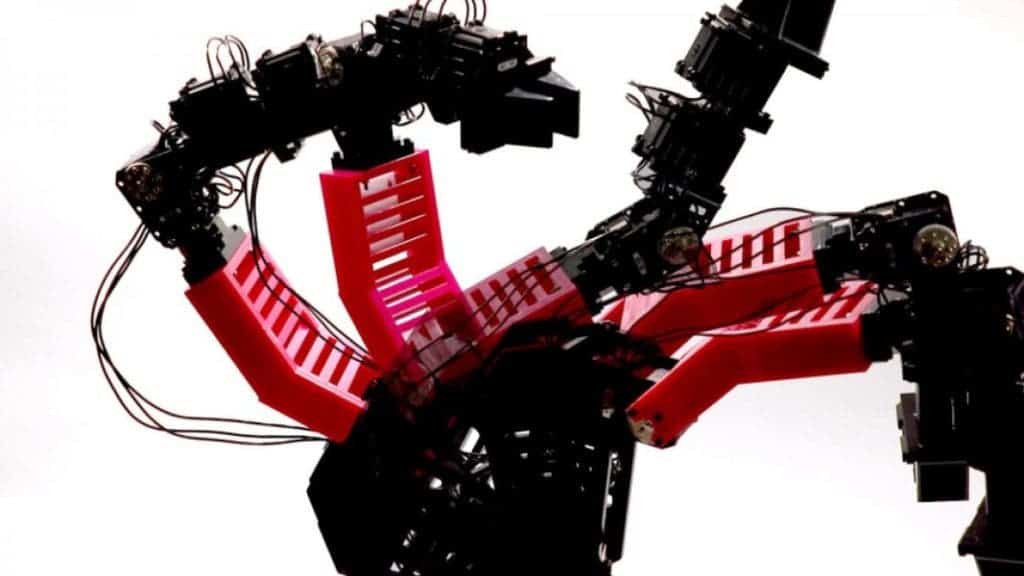
Image credits Robert Kwiatkowski / Columbia Engineering.
Lipson says self-imaging is a cornerstone of taking robots away from “narrow-AI” and towards more general abilities.
But a much more intriguing implication, at least in my eyes, is that the team’s efforts may touch upon the evolutionary origin of our own self-consciousness. The old adage, that teaching is the best way to learn, may apply here — by having to wrestle with machine self-awareness, the team was forced into a clearer view of what gives rise to our own.
“This is perhaps what a newborn child does in its crib, as it learns what it is,” Lipson says. “We conjecture that this advantage may have also been the evolutionary origin of self-awareness in humans. While our robot’s ability to imagine itself is still crude compared to humans, we believe that this ability is on the path to machine self-awareness.”
“Philosophers, psychologists, and cognitive scientists have been pondering the nature self-awareness for millennia, but have made relatively little progress,” he observes. “We still cloak our lack of understanding with subjective terms like ‘canvas of reality,’ but robots now force us to translate these vague notions into concrete algorithms and mechanisms.”
There’s also a darker stroke to this research. Self-awareness would definitely make our robots and associated systems more resilient, adaptable, and overall, versatile. But it “also implies some loss of control,” Lipson and Kwiatkowski warn. “It’s a powerful technology, but it should be handled with care.” The two are not investigating whether bots can be programmed to develop self-models of their ‘mind’ or software, not just their bodies.
The paper “Task-agnostic self-modeling machines” has been published in the journal Science Robotics.
Was this helpful?