
Because they have the potential to reduce our reliance on private cars, ride-sharing apps such as Uber or Lyft intuitively sound like they could solve traffic congestion problems by removing vehicles from the road. But, in reality, things aren’t that simple. According to a new study, ride-sharing might actually make traffic worse in some cities. In San Francisco, for instance, researchers found that these services increased delays by 62% between 2010 and 2016 compared to 22% in a counterfactual 2016 scenario without such services.
Transportation network companies (TNCs) are on-demand ride services where rides are arranged through a mobile app to connect the passenger with the driver of a personal vehicle. In the future, many believe that TNCs will evolve into fleets of self-driving cars that will ferry passengers around the clock.
TNCs have massively grown in their use, accounting for 15% of all intra-San Francisco vehicle trips in 2016 — that’s 12 times the number of taxi trips. Elsewhere, in New York City, TNC ridership equaled that of yellow cabs and doubled annually between 2014 and 2016.
Ride-sharing is often hailed as a solution to traffic congestion problems that plague virtually all major cities around the globe. TNCs may reduce road traffic by shifting trips from personal vehicle to public transit by providing better connections to regional public transportation. Ride-sharing is also a convenient alternative to owning a car, motivating individuals to shift other trips to transit or non-motorized modes (walking, bike, etc.).
However, there are other mechanisms by which ride-sharing might increase traffic congestion. The most obvious contributor is deadheading or the out-of-service period a vehicle has to spend with no passenger. Studies estimate that deadheading is responsible for 50% of TNC traveled miles in New York and 20% in San Francisco. It is also reasonable to believe that many people who would have used public transportation, walked, traveled by bike, or would have made no movement at all are now contributing to traffic congestion by using TNCs because it is so convenient. Finally, TNCs contribute to congestion during the frequent pickups and drop-offs that they have to make — for instance, this behavior causes similar effects to those seen in areas that traditionally rely heavily on taxis.
Researchers at the University of Kentucky and the San Francisco County Transportation Authority wanted to address this debate by comparing traffic congestion in San Francisco with and without the presence of ride-sharing apps in the city. To this aim, they scraped data from the application programming interfaces of Uber and Lyft, along with observational travel times. This allowed the authors to gauge the effect of TNCs on San Francisco’s traffic between 2010 conditions when TNC activity is negligible and 2016 conditions when it is not.
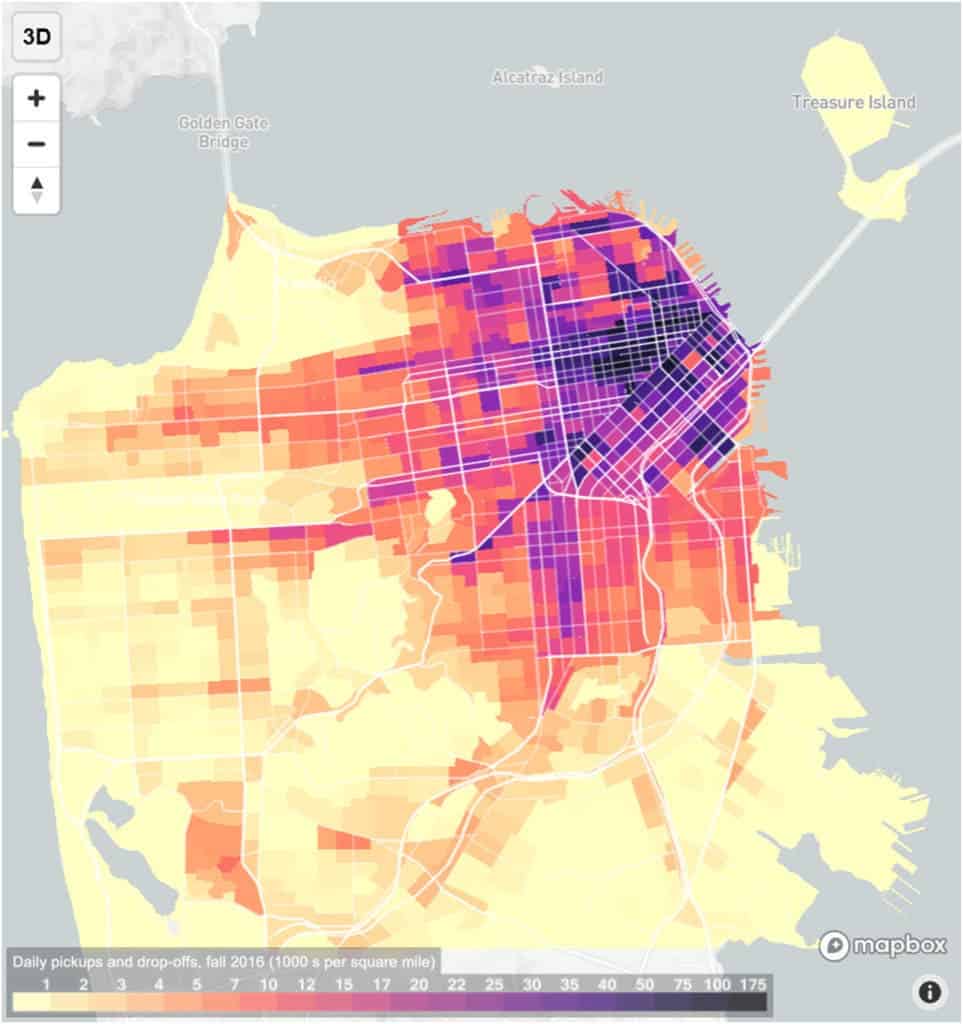
Darker colors represent a higher density of TNC activity). Credit: Science Advances.
In order to exclude other non-TNC factors that contribute to traffic congestion, the authors turned to San Francisco’s travel demand model (SF-CHAMP), which produces estimates of traffic volumes on all roads in San Francisco and is sensitive to changes in population and demographics, employment, transportation networks, and congestion. The version of the model used in this study was calibrated for 2010 conditions, providing a counterfactual case where ride-sharing doesn’t exist.
The results suggest that Uber and Lyft are helping drive more traffic congestion rather than unclogging it. The researchers found that ride-sharing services made delays 62% worse, compared to a 22% increase in travel delays in the scenario where there are no TNCs. The average speed of on-road vehicles decreased by 13% due to TNCs but only 4% in the counterfactual model. Finally, commuters now have to use a longer buffer time to make sure they arrive at their destination on time because travel duration is less reliable overall. According to the findings, this buffer is now 15% higher compared to the natural 6% increase where Uber and Lyft don’t exist.
“The results show some substitution between TNCs and other car trips, but that most TNC trips are adding new cars to the road. The estimated models show that TNC vehicles stopping at the curb to pick up or drop off passengers have a notable disruptive effect on traffic flow, especially on major arterials,” the authors wrote in Science Advances.
The authors say that their findings should be of interest to policy makers and transportation planners who are interested in regulating TNCs in the best interest of the general public of San Francisco. Some solutions include allocating curb spaces and right-of-ways for ride-sharing vehicles and integrating new mobility services.
Was this helpful?