Despite being a relatively new field, image-based machine learning can already accomplish impressive things, but how does imaging analysis could be applied to astronomy? A recent study used machine learning to describe the morphological characteristics of galaxies. The team involved used nearly 27 million galaxies from the Dark Energy Survey (DES) to train, test, and finally implement their method.
What the past has taught us
If we can know the age of each galaxy we observe and know its position, we can better understand how it got there. But cataloging tens and hundreds of millions of galaxies by hand just won’t cut it.
The field of galaxy classification was developed in 1926 by Edwin Hubble. He created a system later called Hubble’s Tuning Fork, which makes it easier to understand the shape and evolution of galaxies.
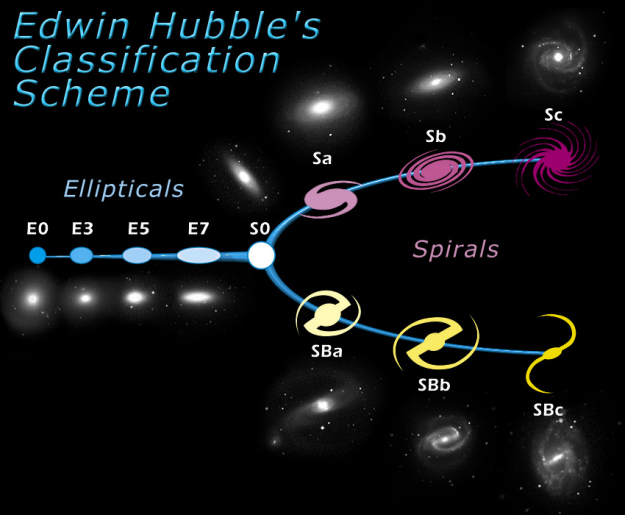
The scheme is shown as a diagram divided into two parts: the elliptical and the spiral galaxies. The elliptical part is classified according to the ellipticity from 0, almost round to 7, very egg-shaped. The spirals are classified by separation of their arms, the tighter the arms are placed around the galaxy bulge it is called ‘a’, when the arms are widely separated it’s called ‘c’. The galaxies’ evolution used to be seen as moving from the left to the right of our fork.
Everything that didn’t fall into these two categories was harder to deal with, making the fork not as general as it strived to be. As astronomy evolved with technology things became more and more complicated. You can see just how complex things become with the image below.
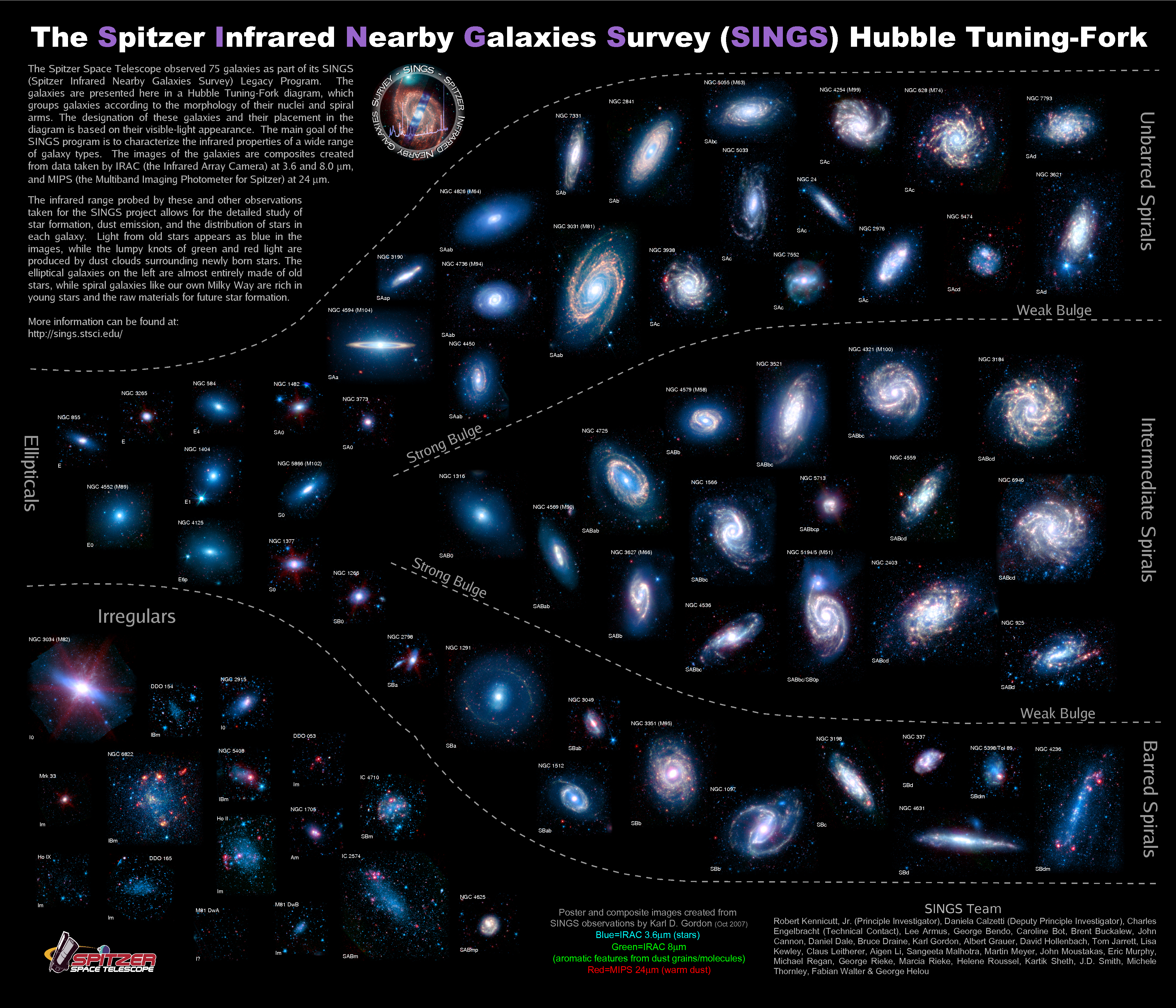
Today we have a large collection of galaxy catalogs with over 2 million galaxies (depending on the catalog). The galaxies not so far away, less faint, are classified by a traditional approach of simply looking at it. Does it look spiral? Yes, then it’s spiral. But there is a problem with that, this is very subjective, and the faint objects can’t be easily classified that easily with our eyes.
Power to the people
A different method was included to try to solve our subjective issue. A citizen science project called Galaxy Zoo classifies galaxies through people’s perspective. Anyone, literally anyone can open its website and start classifying galaxies (yes, you can do it too, and you’d be helping).
In the end, after thousands of people have classified a group of galaxies, astronomers get the results of the ‘poll’ and make statistics with it. The most voted classification wins, a hopefully less subjective result than simply trusting one PhD student for it.
However, this makes scientists’ jobs a lot harder and it gives them more work to deal with. Because before proceeding to more detailed analysis, they need to deal with a large amount of data from people all over the world, losing time and computer efficiency.
Learning like Lieutenant Commander Data
Machine learning is the obvious method for solving the subjectiveness and the amount of data problems. It works like this: you give your computer (or rather, you give a programming language like Python) a bunch of galaxies you know how to classify and teach your computer (model) how to classify them. The code is going to observe all the characteristics of those galaxies and after learning what galaxies can be observed, scientists move to the testing part in which you know nothing of the galaxies and tell the computer to classify. Using some statistics, we can check if the test was a success.
In the end, the whole learning thing is just like our everyday learning. Imagine you have an exam, you read, train with exercises, make mistakes and collect some information in your brain. On the exam date you need to test your knowledge of the things you tried to learn without anything to back you up.
We don’t need to lose time staring at each single galaxy, we don’t have subjective perspectives from one person to the other, and more importantly, it is fast, analysing millions of galaxies in a shorter period of time.
The largest morphology catalog
In the recent study which used DES objects the team trained their model with ~670,000 galaxies which were observed previously by another catalog, the Sloan Digital Sky Server (SDSS). This older catalog already had reliable information necessary to classify galaxies.
After that, they used an astronomical objects simulator, the GALSIM to simulate how galaxies observed by DES would look like if they were fainter. The simulation helped develop the training part of the study. With brighter galaxies, it is easier to classify, so GALSIM would make images with less quality. The result is that the program learns how to predict even the most faint galaxies observed by the catalog. The idea is summarized by the image below.
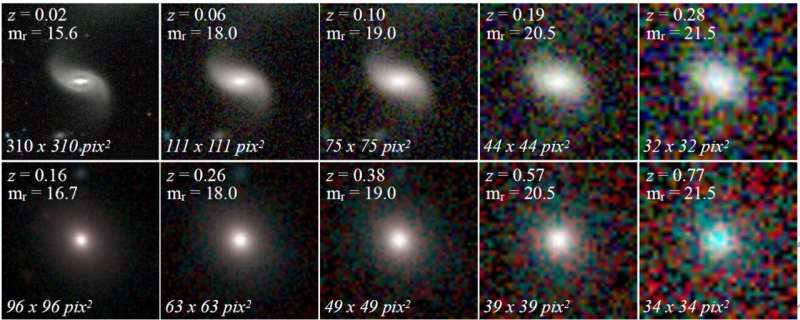
The analysis determined that there has been a 97% accuracy in classifying all those millions of galaxies. This is extremely important for future surveys such as the Legacy Survey of Space and Time (LSST) from the Vera Rubin Observatory. It’s estimated that LSST will be able to observe 20 billion galaxies a year with even fainter objects than DES, no doubt classifying all this would be difficult without machine learning.
The study was published in Monthly Notices of the Royal Astronomical Society.